Common Ground Subjects
The Common Ground for Computing Education consists of broadly-applicable computing classes and curricula for undergraduate and graduate students. Each department or program assesses whether such collaborative classes and curricula make sense for their educational objectives. The Common Ground helps identify potential collaborations, and provides support to departments and programs that find these collaborations beneficial.
Subjects in the Common Ground are identified by “.C” in the subject number. Each course typically has two or more departmental numbers that share the same number after the “C”. For example, Introduction to Computational Science and Engineering is listed under 9.C20, 16.C20, 18.C20, and CSE.C20, showing that four departments (9, 16, 18, and CSE) are collaborating to teach the same subject (C20).
Browse Current Offerings
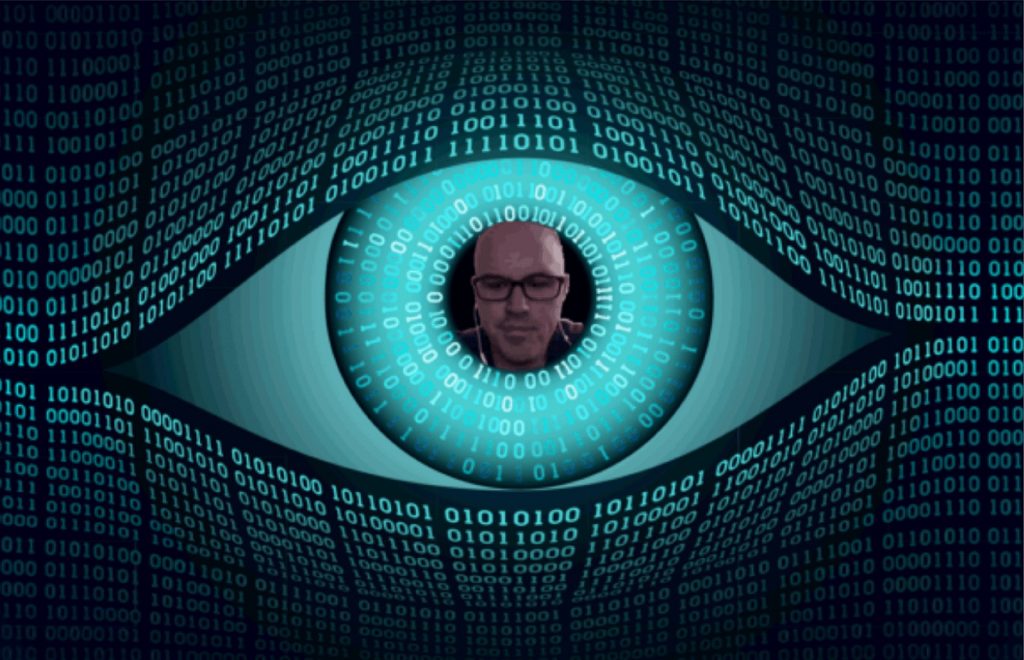
C01/C51 Modeling with Machine Learning: from Algorithms to Applications
Undergraduate and Graduate, 12 units (6+6), spring semester only. Students enroll in the core, 6.C01 or 6.C51, which covers ML fundamentals, plus one discipline-specific module:
- ML for Sustainable Systems
Offered under 1.C01, 1.C51 - Physical Systems Modeling and Design Using ML
Offered under 2.C01, 2.C51 - ML for Molecular Engineering
Offered under 3.C01, 3.C51, 10.C01, 10.C51, 20.C01, 20.C51 - ML in Molecular and Cellular Biology
Offered under 7.C01, 7.C51 - Modeling with ML for CS
Offered under 6.C011, 6.C511 - Modeling with ML: Nuclear Science and Engineering Applications
Offered under 22.C01, 22.C51 - ML Applications for Supply Chain
Offered under SCM.C51
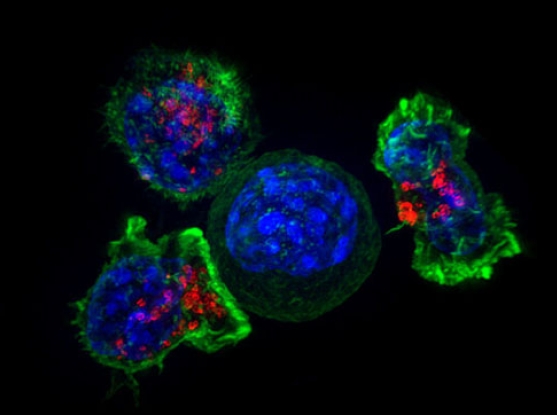
C03 Machine Learning Challenge for Biomedical Discoveries
Undergraduate, 6 units, IAP only, offered under 6.S099 in IAP 2025
Learn more
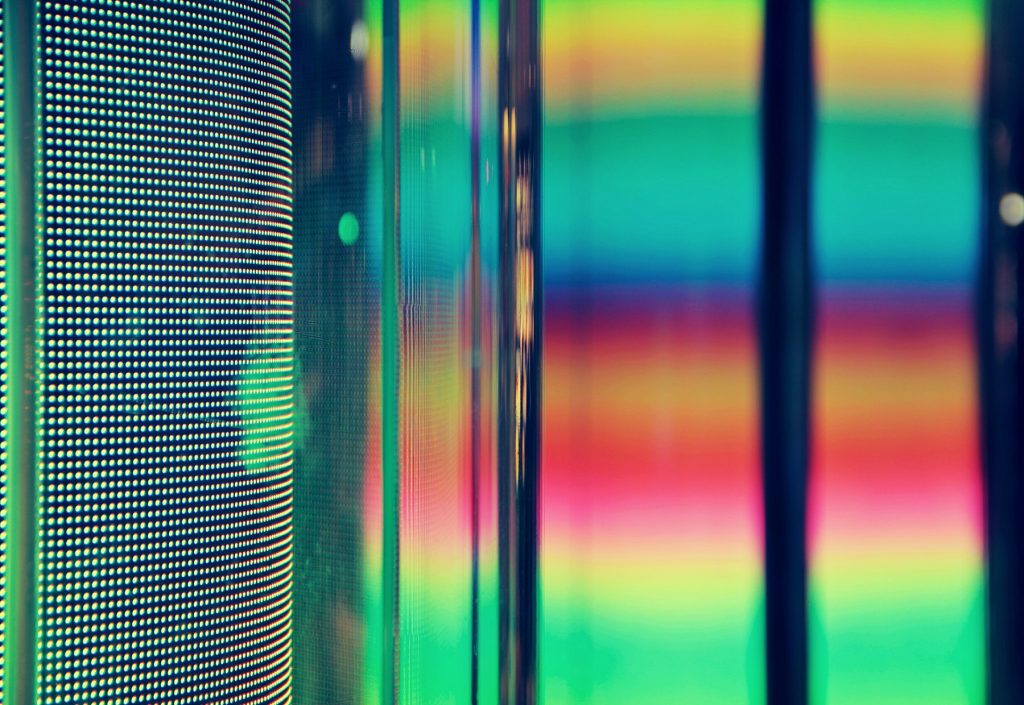
C06 Linear Algebra and Optimization
Undergraduate, 12 units, fall semester only, offered under 6.C06, 18.C06
Learn more
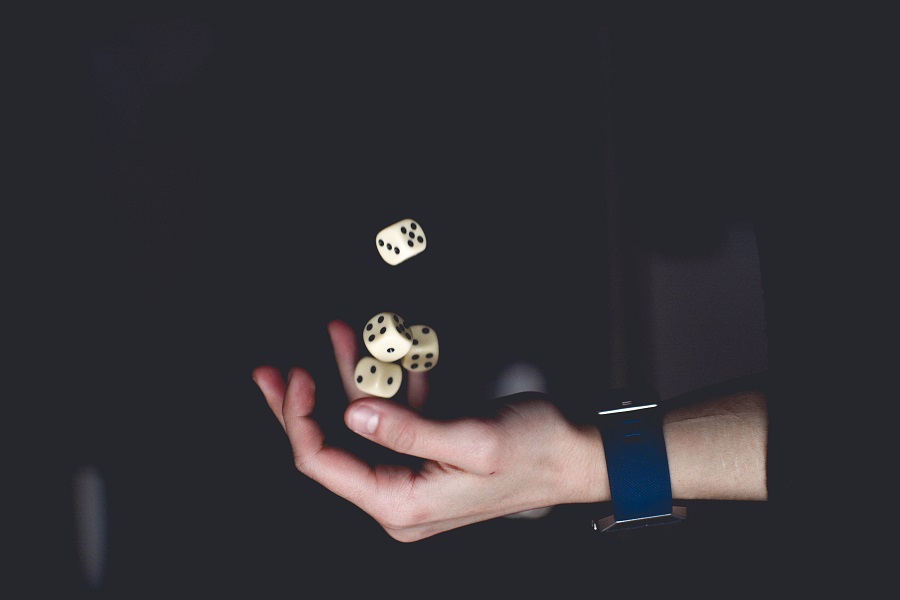
C08 Causal Inference
Undergraduate, 12 units, spring semester only, offered under 15.C08, 17.C08
Learn more
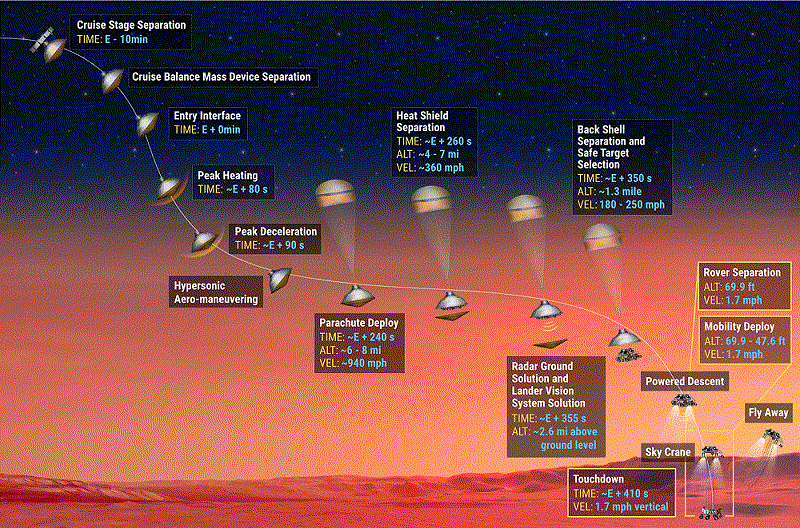
C20 Introduction to Computational Science and Engineering
Undergraduate, 6 units, fall and spring semesters (H2), offered under 9.C20, 16.C20, 18.C20, CSE.C20
Learn more
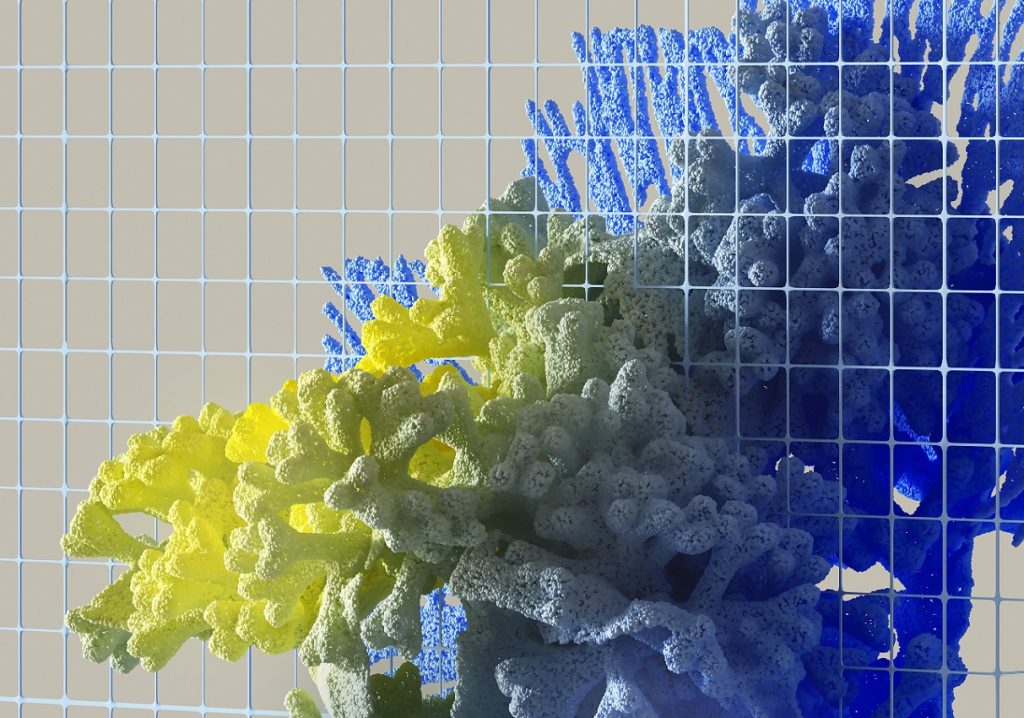
C25 Real World Computation with Julia
Undergraduate, 12 units, fall semester only, offered under 1.C25, 6.C25, 12.C25, 16.C25, 18.C25, 22.C25
Learn more
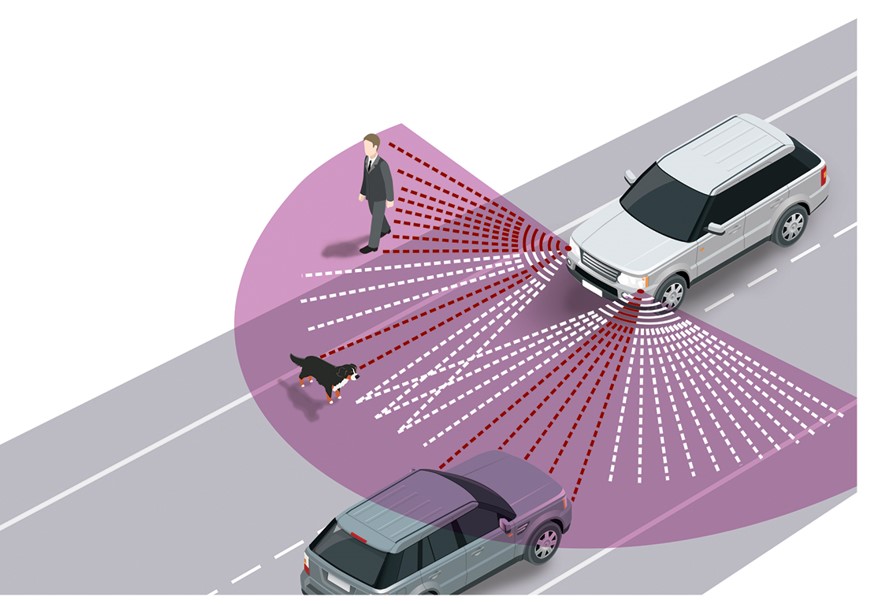
C27/C67 Computational Imaging: Physics and Algorithms
Undergraduate and Graduate, 12 units, fall semester only, offered under 2.C27, 2.C67, 3.C27, 3.C67, 6.C27, 6.C67
Learn more
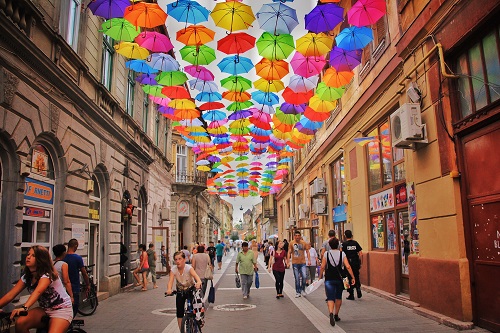
C30 Encoding Culture
Undergraduate, 12 units, spring semester only, offered under 21H.S05 in Spring 2023
Learn more
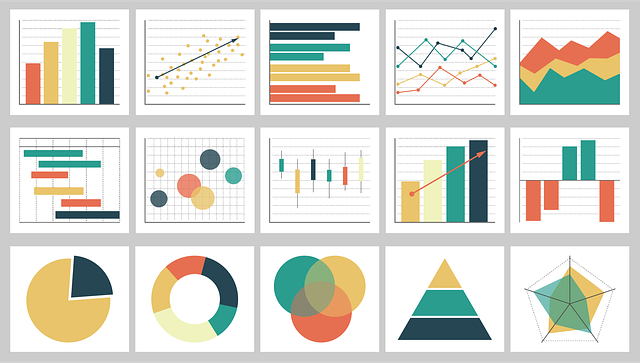
C35/C85 Interactive Data Visualization and Society
Undergraduate and Graduate, 12 units, spring semester only, offered under 6.C35, 6.C85, 11.C35, 11.C85
Learn More
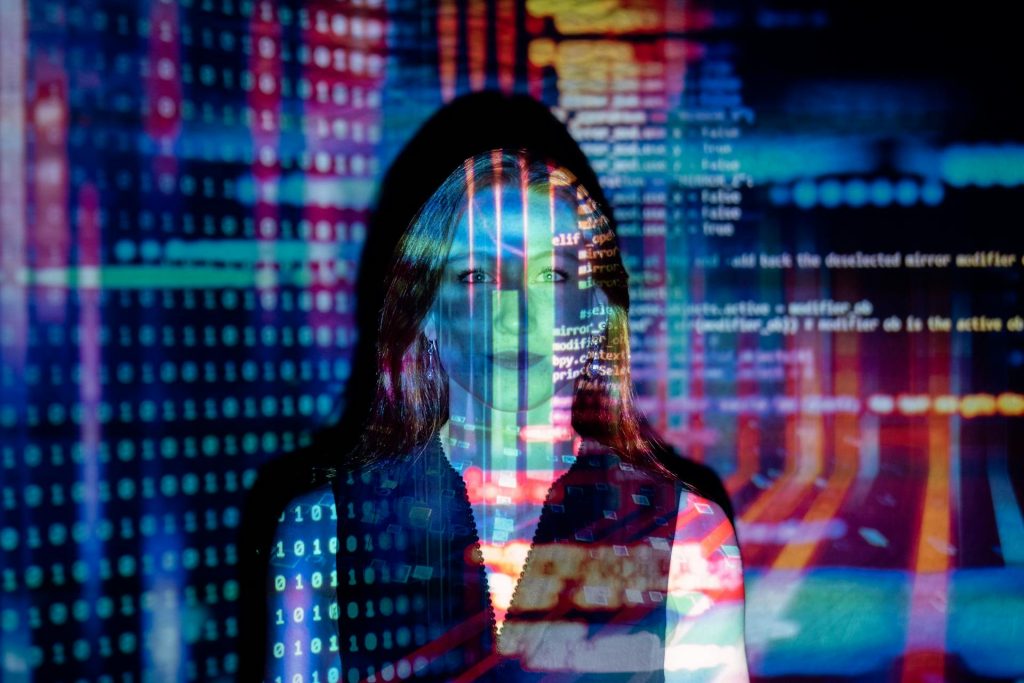
C40 Ethics of Computing
Undergraduate, 12 units, fall semester only, offered under 6.C40 and 24.C40
Learn more
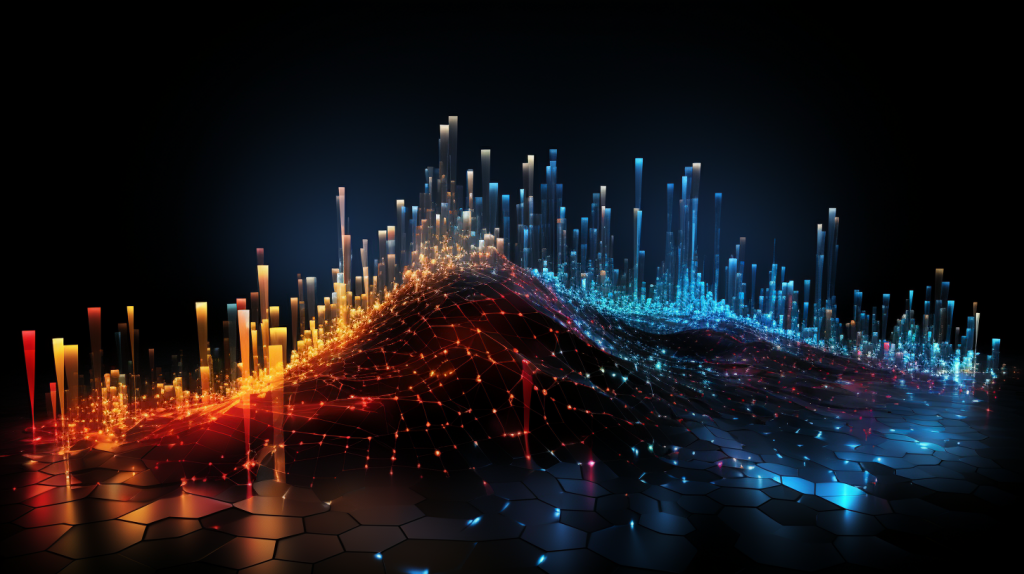
C57 Optimization
Graduate, 12 units, fall semester only, offered under 15.C57, 6.C57, 6.C571, IDS.C57
Learn more