Common Ground for Computing Education
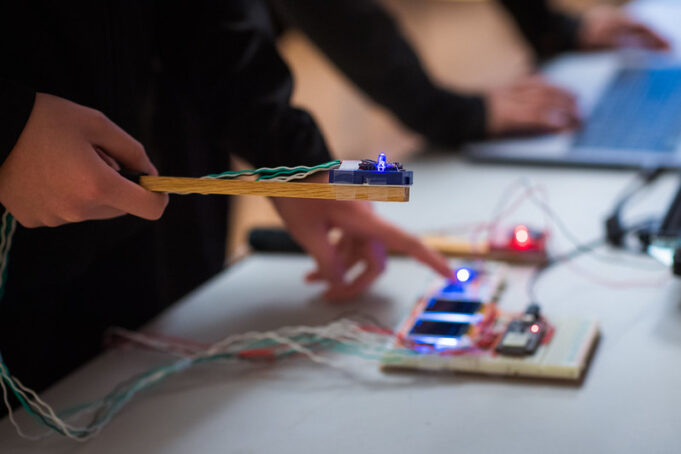
About the Common Ground
Computing permeates every aspect of our lives and impacts education and research across all academic disciplines. The Common Ground capitalizes on this unprecedented opportunity by bringing together experts throughout MIT to facilitate coordinated computing education across the Institute. The initiative brings multiple departments together to develop and teach new courses and launch new programs that blend computing with other disciplines.
Common Ground is led by Deputy Dean for Academics Asu Ozdaglar, the Mathworks Professor of Electrical Engineering and Computer Science and head of the Department of Electrical Engineering and Computer Science (EECS).
Oversight for this initiative is provided by the Common Ground Standing Committee, co-chaired by Ozdaglar and Jeffrey C. Grossman, the Morton and Claire Goulder and Family Professor of Environmental Systems and Professor of of Materials Science and Engineering.
Program Objectives
- Address the need for computing education across many disciplines, not only as a tool but conceptually
- Educate “computing bilinguals”–students fluent in both the “language” of computing and that of their discipline–a core part of the College’s mission
- Bring the forefront of rapidly-changing computer science and artificial intelligence fields together with the problems and methods of other disciplines
- Infuse computing education in a coordinated manner across MIT
Common Ground Subjects
Departments have joined forces to pilot several new subjects under the Common Ground, many of which now satisfy requirements for majors and minors. Some of these subjects include a common core together with discipline-specific material taught in different departments in parallel. Some involve offering variants of existing classes centered around computational thinking in the context of other disciplines. Others create new versions of existing classes with an integrated presentation of computational and numerical tools and issues.
Proposals for new pilots are vetted by the Common Ground Standing Committee. If you have any ideas, please reach out to common-ground@mit.edu.
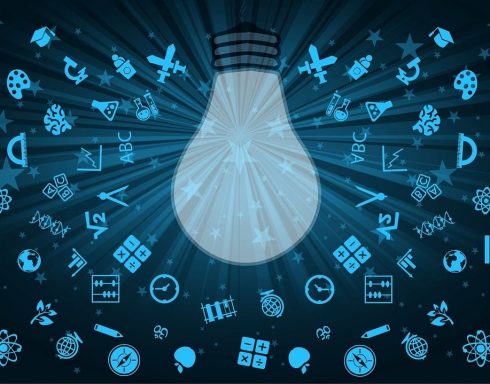