Elevating human health through AI
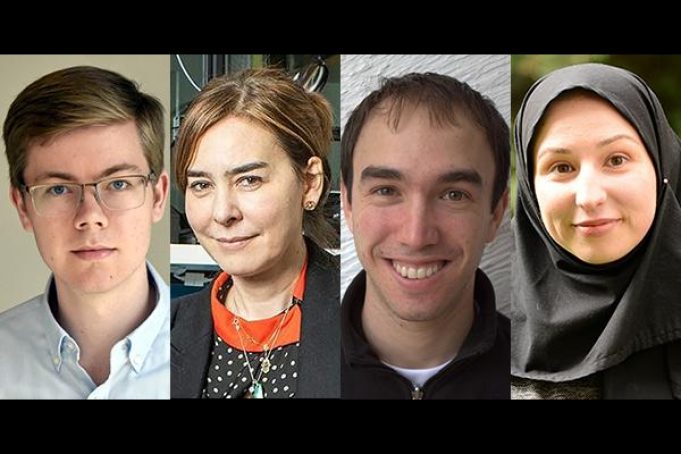
Rapidly improving artificial intelligence (AI) and advanced computing technologies are promising to transform the landscape of human health, from the discovery of biological mechanisms to early disease diagnostics, and from drug discovery to personalized care management.
Building on MIT’s pioneering history in AI and life sciences, researchers in the Stephen A. Schwarzman College of Computing are making new discoveries in machine learning, biology, chemistry, and clinical sciences and translating these discoveries into technologies that can improve people’s lives.
A key area is facilitating new approaches to identifying molecular and biomolecular agents for new medications and new means of diagnosis. Another major area is health care delivery, where novel algorithms, high-performance computing, and machine learning offer new possibilities to transform health monitoring and treatment planning, facilitating better patient care and enabling more effective ways to help prevent disease. In diagnosis, machine-learning methods hold the promise of improved disease detection, increasing both specificity and sensitivity of imaging and testing.
To add to its current expertise in AI and health, the Schwarzman College of Computing is hiring new faculty positions that will be shared between the college and an MIT department or school in six strategic areas, including computing in health and the life sciences. These faculty will help create transformative new computational methods while forging connections with the considerable existing work at MIT.
For example, Dina Katabi SM ’99, PhD ’03, the Thuan and Nicole Pham Professor in the Department of Electrical Engineering and Computer Science (EECS) and principal investigator at MIT’s Abdul Latif Jameel Clinic for Machine Learning in Health (Jameel Clinic) and Computer Science and Artificial Intelligence Laboratory (CSAIL), and her team have developed an AI model that can detect Parkinson’s disease just from reading a person’s breathing patterns.
Katabi demonstrated that the AI assessment of Parkinson’s can be done every night at home while the person is asleep and without touching their body. To do so, the team developed a device with the appearance of a home Wi-Fi router that uses a neural network to discern the presence and severity of one of the fastest-growing neurological diseases in the world.
Katabi notes that the study has important implications for both drug development and clinical care for this disease. “In terms of drug development, the results can enable clinical trials with a significantly shorter duration and fewer participants, ultimately accelerating the development of new therapies. In terms of clinical care, the approach can help in the assessment of Parkinson’s patients in traditionally underserved communities, including those who live in rural areas and those with difficulty leaving home due to limited mobility or cognitive impairment,” she says.
Better Health Records
Buried deep in patients’ electronic health records is a treasure trove of information that could assist in the pursuit to develop highly personalized medicine. Such data, however, are frequently in the form of jargon- and acronym-heavy clinical notes that are inconsistent across health care settings and that have proven difficult for machine-learning models to decipher without time-consuming training and data labeling. This is an obstacle David Sontag SM ’07, PhD ’10 knows all too well.
“It’s challenging to develop a single general-purpose clinical natural language processing system that will solve everyone’s needs and be robust to the huge variation seen across health datasets,” says Sontag, the Hermann L. F. von Helmholtz Professor in EECS and in the Institute for Medical Engineering and Science (IMES).
Today, Sontag, a principal investigator in the Jameel Clinic, CSAIL, and IMES, is leading a group of MIT researchers in leveraging existing powerful large language models—which use massive amounts of internet data to understand and generate human language text—to potentially untangle clinical notes for use in downstream analyses or for live decision support in electronic health records.
The scientists hope to eventually see a single system in place that can extract many types of information, work well at multiple hospitals, and learn from a small amount of labeled data, helping to fulfill the promise of electronic health records to improve care.
“These large language model approaches could potentially transform clinical natural language processing,” Sontag says. “Even if you have hundreds of different use cases, you can build each model with a few minutes of work, versus having to label a ton of data for that particular task.”
Overcoming Bias
“AI, machine learning, and data-driven algorithms in general are only as good as the data they are trained on,” says Marzyeh Ghassemi PhD ’17, who joined IMES and EECS’s Faculty of Artificial Intelligence and Decision-Making (AI+D) in 2021. “We know that biased data create biased models, but equally important is that biased data also create fragile models.”
The Herman L. F. von Helmholtz Career Development Professor explores how these biases play out within the health care system, and how they can be reflected in technologies. For instance, organ transplant allocation systems rely heavily upon risk scores that may be mis-calibrated, and on individual center preferences on operation risk. Addressing such inequities is a central part of Ghassemi’s research goal to create actionable insights in human health that lead to more equitable health care.
Ghassemi, who also holds MIT affiliations with the Jameel Clinic and CSAIL, says her work focuses on neural models that can generate robust clinical knowledge by operating on many modalities of data related to human health. “Unfair and inaccurate predictions can be viewed as a lack of robustness, which has serious consequences in health care,” she notes.
Ghassemi’s investigations also target how the models she is developing can pragmatically be expanded into diverse settings. “Working with the Schwarzman College of Computing brings multidisciplinary interactions to the forefront,” she says, “and enables my group to engage with the multifactorial ways that humans, technology, and AI interact.”
New Molecules, New Therapeutics
At the interface of computer science and chemistry, assistant professor Connor W. Coley SM ’16, PhD ’19 is building his career.
In a shared faculty position between the Department of Chemical Engineering and EECS’s AI+D, Coley, the Henri Slezynger Career Development Professor, develops algorithms and machine-learning systems to streamline the work chemical engineers do in the lab—tools he hopes can accelerate the process of discovering and synthesizing useful molecules.
Working with group members, Coley has built an algorithm-based, machine-learning system, trained on millions of previously published reactions, that analyzes this background information and offers chemists options and suggestions for making molecules. An open-source version of the system has been adopted by chemists and chemical engineers in industry and academia, many of whom conduct pharmaceutical research.
Coley says he navigates “the many, many challenges and failure modes in the development of new therapeutics, from the early stages of target identification to clinical trials.”
“In the discovery phase, one of the challenges is in the identification of therapeutic candidates that exhibit the right balance of properties and appear promising enough to progress to subsequent stages of development,” Coley says.
“The space of possible molecular structures—whether small molecules, peptides, proteins, or one of many other modalities—is enormous and we do not have reliable simulations that can predict how they will function in the complex biological context of the human body.”
Like Ghassemi, Coley is leveraging the highly interdisciplinary Schwarzman College of Computing in his work. “Unique opportunities exist at the interface of disciplines that may not exist when fields are siloed,” he says. “For me, the ability to deeply interact with both chemical engineering and EECS faculty and students has benefitted the work we are able to pursue.”