Design’s new frontier
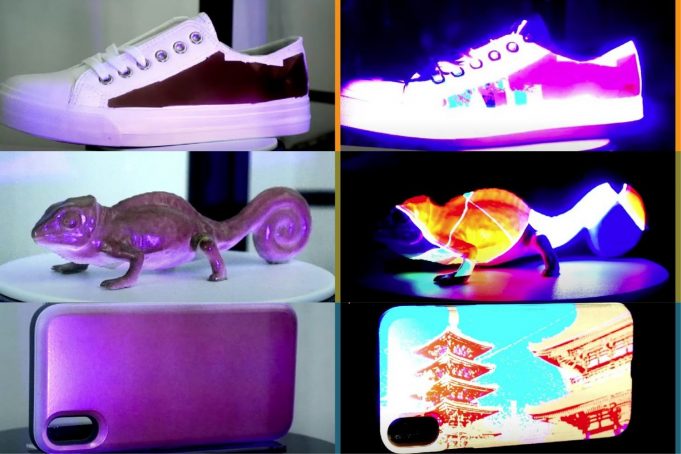
In the 1960s, the advent of computer-aided design (CAD) sparked a revolution in design. For his PhD thesis in 1963, MIT Professor Ivan Sutherland developed Sketchpad, a game-changing software program that enabled users to draw, move, and resize shapes on a computer. Over the course of the next few decades, CAD software reshaped how everything from consumer products to buildings and airplanes were designed.
“CAD was part of the first wave in computing in design. The ability of researchers and practitioners to represent and model designs using computers was a major breakthrough and still is one of the biggest outcomes of design research, in my opinion,” says Maria Yang, Gail E. Kendall Professor and director of MIT’s Ideation Lab.
Innovations in 3D printing during the 1980s and 1990s expanded CAD’s capabilities beyond traditional injection molding and casting methods, providing designers even more flexibility. Designers could sketch, ideate, and develop prototypes or models faster and more efficiently. Meanwhile, with the push of a button, software like that developed by Professor Emeritus David Gossard of MIT’s CAD Lab could solve equations simultaneously to produce a new geometry on the fly.
In recent years, mechanical engineers have expanded the computing tools they use to ideate, design, and prototype. More sophisticated algorithms and the explosion of machine learning and artificial intelligence technologies have sparked a second revolution in design engineering.
Researchers and faculty at MIT’s Department of Mechanical Engineering are utilizing these technologies to re-imagine how the products, systems, and infrastructures we use are designed. These researchers are at the forefront of the new frontier in design.
Computational design
Faez Ahmed wants to reinvent the wheel, or at least the bicycle wheel. He and his team at MIT’s Design Computation & Digital Engineering Lab (DeCoDE) use an artificial intelligence-driven design method that can generate entirely novel and improved designs for a range of products — including the traditional bicycle. They create advanced computational methods to blend human-driven design with simulation-based design.
“The focus of our DeCoDE lab is computational design. We are looking at how we can create machine learning and AI algorithms to help us discover new designs that are optimized based on specific performance parameters,” says Ahmed, an assistant professor of mechanical engineering at MIT.
For their work using AI-driven design for bicycles, Ahmed and his collaborator Professor Daniel Frey wanted to make it easier to design customizable bicycles, and by extension, encourage more people to use bicycles over transportation methods that emit greenhouse gases.
To start, the group gathered a dataset of 4,500 bicycle designs. Using this massive dataset, they tested the limits of what machine learning could do. First, they developed algorithms to group bicycles that looked similar together and explore the design space. They then created machine learning models that could successfully predict what components are key in identifying a bicycle style, such as a road bike versus a mountain bike.
Once the algorithms were good enough at identifying bicycle designs and parts, the team proposed novel machine learning tools that could use this data to create a unique and creative design for a bicycle based on certain performance parameters and rider dimensions.
Ahmed used a generative adversarial network — or GAN — as the basis of this model. GAN models utilize neural networks that can create new designs based on vast amounts of data. However, using GAN models alone would result in homogeneous designs that lack novelty and can’t be assessed in terms of performance. To address these issues in design problems, Ahmed has developed a new method which he calls “PaDGAN,” performance augmented diverse GAN.
“When we apply this type of model, what we see is that we can get large improvements in the diversity, quality, as well as novelty of the designs,” Ahmed explains.
Using this approach, Ahmed’s team developed an open-source computational design tool for bicycles freely available on their lab website. They hope to further develop a set of generalizable tools that can be used across industries and products.
Longer term, Ahmed has his sights set on loftier goals. He hopes the computational design tools he develops could lead to “design democratization,” putting more power in the hands of the end user.
“With these algorithms, you can have more individualization where the algorithm assists a customer in understanding their needs and helps them create a product that satisfies their exact requirements,” he adds.
Using algorithms to democratize the design process is a goal shared by Stefanie Mueller, an associate professor in electrical engineering and computer science and mechanical engineering.
Personal fabrication
Platforms like Instagram give users the freedom to instantly edit their photographs or videos using filters. In one click, users can alter the palette, tone, and brightness of their content by applying filters that range from bold colors to sepia-toned or black-and-white. Mueller, X-Window Consortium Career Development Professor, wants to bring this concept of the Instagram filter to the physical world.
“We want to explore how digital capabilities can be applied to tangible objects. Our goal is to bring reprogrammable appearance to the physical world,” explains Mueller, director of the HCI Engineering Group based out of MIT’s Computer Science and Artificial Intelligence Laboratory.
Mueller’s team utilizes a combination of smart materials, optics, and computation to advance personal fabrication technologies that would allow end users to alter the design and appearance of the products they own. They tested this concept in a project they dubbed “Photo-Chromeleon.”
First, a mix of photochromic cyan, magenta, and yellow dies are airbrushed onto an object — in this instance, a 3D sculpture of a chameleon. Using software they developed, the team sketches the exact color pattern they want to achieve on the object itself. An ultraviolet light shines on the object to activate the dyes.
To actually create the physical pattern on the object, Mueller has developed an optimization algorithm to use alongside a normal office projector outfitted with red, green, and blue LED lights. These lights shine on specific pixels on the object for a given period of time to physically change the makeup of the photochromic pigments.
“This fancy algorithm tells us exactly how long we have to shine the red, green, and blue light on every single pixel of an object to get the exact pattern we’ve programmed in our software,” says Mueller.
Giving this freedom to the end user enables limitless possibilities. Mueller’s team has applied this technology to iPhone cases, shoes, and even cars. In the case of shoes, Mueller envisions a shoebox embedded with UV and LED light projectors. Users could put their shoes in the box overnight and the next day have a pair of shoes in a completely new pattern.
Mueller wants to expand her personal fabrication methods to the clothes we wear. Rather than utilize the light projection technique developed in the PhotoChromeleon project, her team is exploring the possibility of weaving LEDs directly into clothing fibers, allowing people to change their shirt’s appearance as they wear it. These personal fabrication technologies could completely alter consumer habits.
“It’s very interesting for me to think about how these computational techniques will change product design on a high level,” adds Mueller. “In the future, a consumer could buy a blank iPhone case and update the design on a weekly or daily basis.”
Computational fluid dynamics and participatory design
Another team of mechanical engineers, including Sili Deng, the Brit (1961) & Alex (1949) d’Arbeloff Career Development Professor, are developing a different kind of design tool that could have a large impact on individuals in low- and middle-income countries across the world.
As Deng walked down the hallway of Building 1 on MIT’s campus, a monitor playing a video caught her eye. The video featured work done by mechanical engineers and MIT D-Lab on developing cleaner burning briquettes for cookstoves in Uganda. Deng immediately knew she wanted to get involved.
“As a combustion scientist, I’ve always wanted to work on such a tangible real-world problem, but the field of combustion tends to focus more heavily on the academic side of things,” explains Deng.
After reaching out to colleagues in MIT D-Lab, Deng joined a collaborative effort to develop a new cookstove design tool for the 3 billion people across the world who burn solid fuels to cook and heat their homes. These stoves often emit soot and carbon monoxide, leading not only to millions of deaths each year, but also worsening the world’s greenhouse gas emission problem.
The team is taking a three-pronged approach to developing this solution, using a combination of participatory design, physical modeling, and experimental validation to create a tool that will lead to the production of high-performing, low-cost energy products.
Deng and her team in the Deng Energy and Nanotechnology Group use physics-based modeling for the combustion and emission process in cookstoves.
“My team is focused on computational fluid dynamics. We use computational and numerical studies to understand the flow field where the fuel is burned and releases heat,” says Deng.
These flow mechanics are crucial to understanding how to minimize heat loss and make cookstoves more efficient, as well as learning how dangerous pollutants are formed and released in the process.
Using computational methods, Deng’s team performs three-dimensional simulations of the complex chemistry and transport coupling at play in the combustion and emission processes. They then use these simulations to build a combustion model for how fuel is burned and a pollution model that predicts carbon monoxide emissions.
Deng’s models are used by a group led by Daniel Sweeney in MIT D-Lab to test the experimental validation in prototypes of stoves. Finally, Professor Maria Yang uses participatory design methods to integrate user feedback, ensuring the design tool can actually be used by people across the world.
The end goal for this collaborative team is to not only provide local manufacturers with a prototype they could produce themselves, but to also provide them with a tool that can tweak the design based on local needs and available materials.
Deng sees wide-ranging applications for the computational fluid dynamics her team is developing.
“We see an opportunity to use physics-based modeling, augmented with a machine learning approach, to come up with chemical models for practical fuels that help us better understand combustion. Therefore, we can design new methods to minimize carbon emissions,” she adds.
While Deng is utilizing simulations and machine learning at the molecular level to improve designs, others are taking a more macro approach.
Designing intelligent systems
When it comes to intelligent design, Navid Azizan thinks big. He hopes to help create future intelligent systems that are capable of making decisions autonomously by using the enormous amounts of data emerging from the physical world. From smart robots and autonomous vehicles to smart power grids and smart cities, Azizan focuses on the analysis, design, and control of intelligent systems.
Achieving such massive feats takes a truly interdisciplinary approach that draws upon various fields such as machine learning, dynamical systems, control, optimization, statistics, and network science, among others.
“Developing intelligent systems is a multifaceted problem, and it really requires a confluence of disciplines,” says Azizan, assistant professor of mechanical engineering with a dual appointment in MIT’s Institute for Data, Systems, and Society (IDSS). “To create such systems, we need to go beyond standard approaches to machine learning, such as those commonly used in computer vision, and devise algorithms that can enable safe, efficient, real-time decision-making for physical systems.”
For robot control to work in the complex dynamic environments that arise in the real world, real-time adaptation is key. If, for example, an autonomous vehicle is going to drive in icy conditions or a drone is operating in windy conditions, they need to be able to adapt to their new environment quickly.
To address this challenge, Azizan and his collaborators at MIT and Stanford University have developed a new algorithm that combines adaptive control, a powerful methodology from control theory, with meta learning, a new machine learning paradigm.
“This ‘control-oriented’ learning approach outperforms the existing ‘regression-oriented’ methods, which are mostly focused on just fitting the data, by a wide margin,” says Azizan.
Another critical aspect of deploying machine learning algorithms in physical systems that Azizan and his team hope to address is safety. Deep neural networks are a crucial part of autonomous systems. They are used for interpreting complex visual inputs and making data-driven predictions of future behavior in real time. However, Azizan urges caution.
“These deep neural networks are only as good as their training data, and their predictions can often be untrustworthy in scenarios not covered by their training data,” he says. Making decisions based on such untrustworthy predictions could lead to fatal accidents in autonomous vehicles or other safety-critical systems.
To avoid these potentially catastrophic events, Azizan proposes that it is imperative to equip neural networks with a measure of their uncertainty. When the uncertainty is high, they can then be switched to a “safe policy.”
In pursuit of this goal, Azizan and his collaborators have developed a new algorithm known as SCOD — Sketching Curvature of Out-of-Distribution Detection. This framework could be embedded within any deep neural network to equip them with a measure of their uncertainty.
“This algorithm is model-agnostic and can be applied to neural networks used in various kinds of autonomous systems, whether it’s drones, vehicles, or robots,” says Azizan.
Azizan hopes to continue working on algorithms for even larger-scale systems. He and his team are designing efficient algorithms to better control supply and demand in smart energy grids. According to Azizan, even if we create the most efficient solar panels and batteries, we can never achieve a sustainable grid powered by renewable resources without the right control mechanisms.
Mechanical engineers like Ahmed, Mueller, Deng, and Azizan serve as the key to realizing the next revolution of computing in design.
“MechE is in a unique position at the intersection of the computational and physical worlds,” Azizan says. “Mechanical engineers build a bridge between theoretical, algorithmic tools and real, physical world applications.”
Sophisticated computational tools, coupled with the ground truth mechanical engineers have in the physical world, could unlock limitless possibilities for design engineering, well beyond what could have been imagined in those early days of CAD.