Creating a versatile vaccine to take on Covid-19 in its many guises
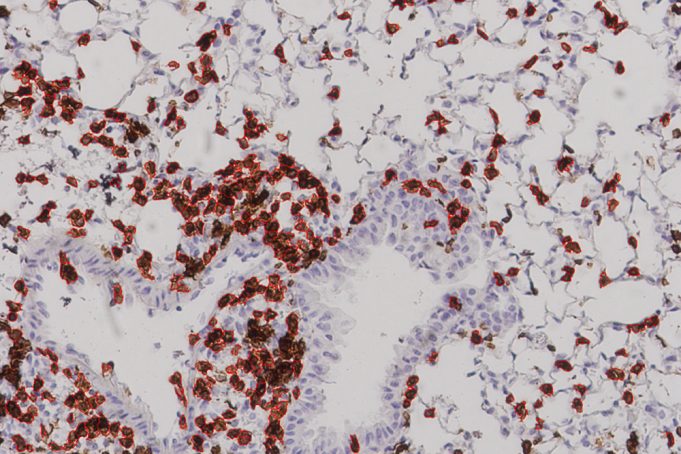
Aided by machine learning, scientists are working to develop a vaccine that would be effective against all SARS-Cov-2 strains.
One of the 12 labors of Hercules, according to ancient lore, was to destroy a nine-headed monster called the Hydra. The challenge was that when Hercules used his sword to chop off one of the monster’s heads, two would grow back in its place. He therefore needed an additional weapon, a torch, to vanquish his foe.
There are parallels between this legend and our three-years-and-counting battle with SARS-Cov-2, the virus that causes Covid-19. Every time scientists have thought they’d subdued one strain of the virus — be it alpha, beta, delta, or omicron — another variant or subvariant emerged a short while later.
For this reason, researchers at MIT and other institutions are preparing a new strategy against the virus — a novel vaccine that, unlike those in use today, could potentially counteract all variants of the disease, having a property called “pan-variance” that could circumvent the need for a different booster shot every time a new strain comes into circulation. In a paper published today in the journal Frontiers in Immunology, the team reports on experiments with mice that demonstrate the vaccine’s effectiveness in preventing death from Covid-19 infection.
Viral vaccines typically work by exposing the immune system to a small piece of the virus. That can create learned responses that protect people later when they’re exposed to the actual virus. The premise of standard Covid-19 vaccines, such as those produced by Moderna and Pfizer, is to activate the part of the immune system that releases neutralizing antibodies. They do this by providing cells with instructions (in the form of mRNA molecules) for making the spike protein — a protein found on the surface of the Covid-19 virus whose presence can trigger an immune reaction. “The problem with that approach is that the target keeps changing” — the spike protein itself can vary among different viral strains — “and that can make the vaccine ineffective,” says David Gifford, an MIT professor in electrical engineering and computer science and biological engineering, as well as a coauthor of the Frontiers paper.
He and his colleagues, accordingly, have taken a different approach, selecting a different target for their vaccine: activating the part of the immune system that unleashes “killer” T cells, which attack cells infected with the virus. A vaccine of this sort will not keep people from getting Covid-19, but it could keep them from getting very sick or dying.
A key innovation made by this group — which included researchers from MIT, the University of Texas, Boston University, Tufts University, Massachusetts General Hospital, and Acuitas Therapeutics — was to bring machine learning techniques into the vaccine design process. A critical aspect of that process involves determining which parts of SARS-Cov-2, which peptides (chains of amino acids that are the building blocks of proteins), should go into the vaccine. That entails sifting through thousands of peptides in the virus and picking out just 30 or so that should be incorporated.
But that decision has to take into account so-called HLA molecules — protein fragments on the surface of cells that serve as “billboards,” telling immune cells (which lack X-ray vision) what is going on inside other cells. The display of specific protein fragments can indicate, for instance, that a certain cell is infected by SARS-Cov-2 and should be gotten rid of.
Machine learning algorithms were used to solve a complicated set of “optimization problems,” notes Brandon Carter, a PhD student in MIT’s Department of Electrical Engineering and Computer Science, an affiliate of the MIT Computer Science and Artificial Intelligence Laboratory (CSAIL), and a lead author of the new paper. The overriding goal is to select peptides that are present, or “conserved,” in all variants of the virus. But those peptides also need to be associated with HLA molecules that have a high likelihood of being displayed so they can alert the immune system. “You want this to happen in as many people as possible to get maximum population coverage from your vaccine,” Carter says. Furthermore, you want each individual to be covered multiple times by the vaccine, he adds. “This means that more than one peptide in the vaccine is predicted to be displayed by some HLA in each person.” Achieving these various objectives is a task that can be significantly expedited by machine learning tools.
While that touches on the theoretical end of this project, the latest results came from experiments carried out by collaborators at the University of Texas Medical Branch in Galveston, which showed a strong immune response in mice given the vaccine. The mice in this experiment did not die but were were “humanized,” meaning that they had an HLA molecule found in human cells. “This study,” Carter says, “offers proof in a living system, an actual mouse, that the vaccines we devised using machine learning can afford protection from the Covid virus.” Gifford characterizes their work as “the first experimental evidence that a vaccine formulated in this fashion would be effective.”
Paul Offit, a professor of pediatrics in the Division of Infectious Diseases at Children’s Hospital of Philadelphia, finds the results encouraging. “A lot of people wonder about what approaches will be used to make Covid-19 vaccines in the future,” Offit says. “Given that T cells are critical in protection against severe Covid-19, future vaccines that focus on inducing the broadest T cell responses will be an important step forward in the next generation of vaccines.”
More animal studies — and eventual human studies — would have to be done before this work can usher in the “next generation of vaccines.” The fact that 24 percent of the lung cells in vaccinated mice were T cells, Gifford says, “showed that their immune systems were poised to fight viral infection.” But one has to be careful to avoid too strong of an immune response, he cautions, so as not to cause lung damage.
Other questions abound. Should T-cell vaccines be used instead of, or in combination with, standard spike protein vaccines? While it might be possible to enhance existing vaccines by including a T-cell component, Gifford says, “putting two things together may not be strictly additive, as one part of the vaccine could mask the other.”
Nevertheless, he and his colleagues believe their T-cell vaccine has the potential to help immunocompromised individuals who cannot produce neutralizing antibodies and thus may not benefit from traditional Covid vaccines. Their vaccine may also alleviate suffering from “long Covid” in people who continue to harbor reservoirs of the virus well after their initial infection.
The mechanism behind current flu vaccines, like current Covid-19 vaccines, is to induce neutralizing antibodies, but those vaccines don’t always work for different influenza strains. Carter sees potential for flu vaccines based on a T-cell response, “which may prove to be more effective, providing broader coverage, because of their pan-variance.”
Nor are the methods they are developing limited to Covid-19 or the flu, he maintains, as they might someday be applied to cancer. Gifford agrees, saying that a T-cell vaccine — designed to maximize immune protection both within an individual and among the greatest number of individuals — could become a key asset in the fight against cancer. “That’s not within the scope of our present study,” he says, “but it could be the subject of future work.”
Other MIT contributors to the work were Ge Liu and Alexander Dimitrakakis. The work was supported, in part, by Schmidt Futures and a C3.ai grant to David Gifford.