Behind Covid-19 vaccine development
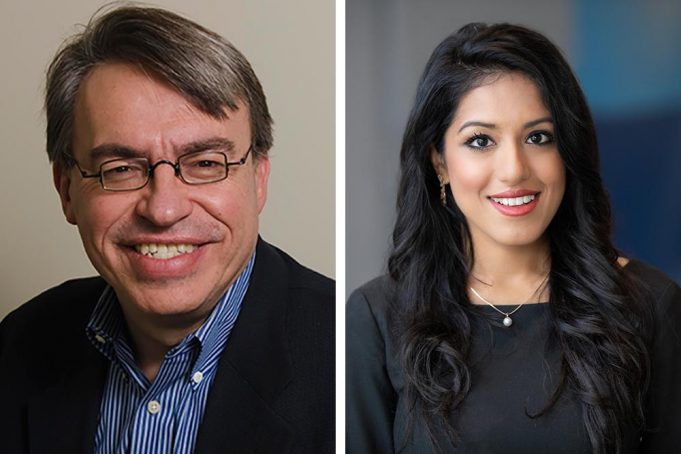
A machine learning model developed jointly by Janssen and MIT data scientists played a key role in the clinical trial process for the Johnson & Johnson Covid-19 vaccine.
When starting a vaccine program, scientists generally have anecdotal understanding of the disease they’re aiming to target. When Covid-19 surfaced over a year ago, there were so many unknowns about the fast-moving virus that scientists had to act quickly and rely on new methods and techniques just to even begin understanding the basics of the disease.
Scientists at Janssen Research & Development, developers of the Johnson & Johnson Covid-19 vaccine, leveraged real-world data and, working with MIT researchers, applied artificial intelligence and machine learning to help guide the company’s research efforts into a potential vaccine.
“Data science and machine learning can be used to augment scientific understanding of a disease,” says Najat Khan, chief data science officer and global head of strategy and operations for Janssen Research & Development. “For Covid-19, these tools became even more important because our knowledge was rather limited. There was no hypothesis at the time. We were developing an unbiased understanding of the disease based on real-world data using sophisticated AI/ML algorithms.”
In preparing for clinical studies of Janssen’s lead vaccine candidate, Khan put out a call for collaborators on predictive modeling efforts to partner with her data science team to identify key locations to set up trial sites. Through Regina Barzilay, the MIT School of Engineering Distinguished Professor for AI and Health, faculty lead of AI for MIT’s Abdul Latif Jameel Clinic for Machine Learning in Health, and a member of Janssen’s scientific advisory board, Khan connected with Dimitris Bertsimas, the Boeing Leaders for Global Operations Professor of Management at MIT Sloan School of Management, who had developed a leading machine learning model that tracks Covid-19 spread in communities and predicts patient outcomes, and brought him on as the primary technical partner on the project.
DELPHI
When the World Health Organization declared Covid-19 a pandemic in March 2020 and forced much of the world into lockdown, Bertsimas, who is also the faculty lead of entrepreneurship for the Jameel Clinic, brought his group of 25-plus doctoral and master’s students together to discuss how they could use their collective skills in machine learning and optimization to create new tools to aid the world in combating the spread of the disease.
The group started tracking their efforts on the COVIDAnalytics platform, where their models are generating accurate real-time insight into the pandemic. One of the group’s first projects was charting the progression of Covid-19 with an epidemiological model they developed named DELPHI, which predicts state-by-state infection and mortality rates based upon each state’s policy decision.
DELPHI is based on the standard SEIR model, a compartmental model that simplifies the mathematical modeling of infectious diseases by dividing populations in four categories: susceptible, exposed, infectious, and recovered. The ordering of the labels is intentional to show the flow patterns between the compartments. DELPHI expands on this model with a system that looks at 11 possible states of being to account for realistic effects of the pandemic, such comparing the length of time those who recovered from Covid-19 spent in the hospital versus those who died.
“The model has some values that are hardwired, such as how long a person stays in the hospital, but we went deeper to account for the nonlinear change of infection rates, which we found were not constant and varied over different periods and locations,” says Bertsimas. “This gave us more modeling flexibility, which led the model to make more accurate predictions.”
A key innovation of the model is capturing the behaviors of people related to measures put into place during the pandemic, such as lockdowns, mask-wearing, and social distancing, and the impact these had on infection rates.
“By June or July, we were able to augment the model with these data. The model then became even more accurate,” says Bertsimas. “We also considered different scenarios for how various governments might respond with policy decisions, from implementing serious restrictions to no restrictions at all, and compared them to what we were seeing happening in the world. This gave us the ability to make a spectrum of predictions. One of the advantages of the DELPHI model is that it makes predictions on 120 countries and all 50 U.S. states on a daily basis.”
A vaccine for today’s pandemic
Being able to determine where Covid-19 is likely to spike next proved to be critical to the success of Janssen’s clinical trials, which were “event-based” — meaning that “we figure out efficacy based on how many ‘events’ are in our study population, events such as becoming sick with Covid-19,” explains Khan.
“To run a trial like this, which is very, very large, it’s important to go to hot spots where we anticipate the disease transmission to be high so that you can accumulate those events quickly. If you can, then you can run the trial faster, bring the vaccine to market more quickly, and also, most importantly, have a very rich dataset where you can make statistically sound analysis.”
Bertsimas assembled a core group of researchers to work with him on the project, including two doctoral students from MIT’s Operations Research Center, where he is a faculty member: Michael Li, who led implementation efforts, and Omar Skali Lami. Other members included Hamza Tazi Bouardi MBN ’20, a former master of business analytics student, and Ali Haddad, a data research scientist at Dynamic Ideas LLC.
The MIT team began collaborating with Khan and her team last May to forecast where the next surge in cases might happen. Their goal was to identify Covid-19 hot spots where Janssen could conduct clinical trials and recruit participants who were most likely to get exposed to the virus.
With clinical trials due to start last September, the teams had to immediately hit the ground running and make predictions four months in advance of when the trials would actually take place. “We started meeting daily with the Janssen team. I’m not exaggerating — we met on a daily basis … sometimes over the weekend, and sometimes more than once a day,” says Bertsimas.
To understand how the virus was moving around the world, data scientists at Janssen continuously monitored and scouted data sources across the world. The team built a global surveillance dashboard that pulled in data at a country, state, and even county level based on data availability, on case numbers, hospitalizations, and mortality and testing rates.
The DELPHI model integrated these data, with additional information about local policies and behaviors, such as whether people were being compliant with mask-wearing, and was making daily predictions in the 300-400 range. “We were getting constant feedback from the Janssen team which helped to improve the quality of the model. The model eventually became quite central to the clinical trial process,” says Bertsimas.
Remarkably, the vast majority of Janssen’s clinical trial sites that DELPHI predicted to be Covid-19 hot spots ultimately had extremely high number of cases, including in South Africa and Brazil, where new variants of the virus had surfaced by the time the trials began. According to Khan, high incidence rates typically indicate variant involvement.
“All of the predictions the model made are publicly available, so one can go back and see how accurate the model really is. It held its own. To this day, DELPHI is one of the most accurate models the scientific community has produced,” says Bertsimas.
“As a result of this model, we were able to have a highly data-rich package at the time of submission of our vaccine candidate,” says Khan. “We are one of the few trials that had clinical data in South Africa and Brazil. That became critical because we were able to develop a vaccine that became relevant for today’s needs, today’s world, and today’s pandemic, which consists of so many variants, unfortunately.”
Khan points out that the DELPHI model was further evolved with diversity in mind, taking into account biological risk factors, patient demographics, and other characteristics. “Covid-19 impacts people in different ways, so it was important to go to areas where we were able to recruit participants from different races, ethnic groups, and genders. Due to this effort, we had one of the most diverse Covid-19 trials that’s been run to date,” she says. “If you start with the right data, unbiased, and go to the right places, we can actually change a lot of the paradigms that are limiting us today.”
In April, the MIT and Janssen R&D Data Science team were jointly recognized by the Institute for Operations Research and the Management Sciences (INFORMS) as the winner of the 2021 Innovative Applications in Analytics Award for their innovative and highly impactful work on Covid-19. Building on this success, the teams are continuing their collaboration to apply their data-driven approach and technical rigor in tackling other infectious diseases. “This was not a partnership in name only. Our teams really came together in this and continue to work together on various data science efforts across the pipeline,” says Khan. The team further appreciates the role of investigators on the ground, who contributed to site selection in combination with the model.
“It was a very satisfying experience,” concurs Bertsimas. “I’m proud to have contributed to this effort and help the world in the fight against the pandemic.